Determining the likelihood and probability of risks can be quite subjective. Here’s how you can change that.
Not all organizational risks are catastrophic. Some risks, like employee turnover, are a daily operational issue that just need to be managed. But other risks, like a data breach, can be very difficult to mitigate and cause irreversible damage.
So, in a massive organization with many controls across different areas, it’s only logical to focus on the most critical risks—those that have the highest impact on the organization or a high probability of occurring.
But even the terms “impact” and “probability” are highly subjective. Ask five different people and they’ll have five different opinions.
“… eliminate the “how likely is this?” debate by digging into the data to see if and when the risk happened in the past.”
This is where analytics and data come into the equation. Analytics connect to your organization’s data sources to analyze 100% of your data and work to quantify risk. This helps remove the subjectivity.
You can eliminate the “how likely is this?” debate by digging into the data to see if and when the risk happened in the past. And in some cases you can quantify the bottom line impact. If the risk occurred in the past, you can use the data to uncover the direct and indirect costs of the error, plus the fix to end up with a dollar figure. Now, instead of a subjective assumption, you have a data-driven answer for how likely a risk is, as well as its financial impact. With analytics, your classification of low, medium, or high is no longer subjective, but backed up by data.
An example of how data helps remove subjectivity
Acme Inc. had a number of ex-employees with active IDs in their SAP financial reporting system—a risk that many organizations face. Acme saw this risk as low because the organization made sure to collect people’s building access cards when they left the company and removed ex-employee network access, so they couldn’t access SAP.
Acme’s external audit firm saw the situation quite differently. It categorized the risk as high on the basis that ex-employees might still be able to access the system if they’d shared passwords before.
So Acme looked to their data to help solve this impasse.
First, they ran a test to identify former employees who still had access to SAP, and see if any IDs were used after the date of employment termination. This test was done to tell them the “likelihood” of this risk/ and whether it had happened in the past.
Second, they looked at the activities undertaken by those IDs, telling them the “impact.” Now, Acme could talk facts instead of assumptions. The company was able to agree on an appropriate course of action.
Acme and their external auditors might have spent weeks debating the scoring of this risk without any progress. This is because both arguments were based on subjective assumptions. Instead, using a fairly simple set of analytics, they were able to quantify the exposure in a way that no one could argue with.
This is just one example of how data can drive efficiency and confident decision-making.
Of course, this doesn’t apply to all risks (e.g., risks that have not happened in the past but may in the future, like a water shortage in a key supplier region). Regardless, analytics can always be used to supplement the subjectivity of the risk assessment process, and add facts to areas where you also need to make educated guesses.
Learn more about how RiskBond can help you identify, assesses, respond to and monitor your enterprise risks.
eBook
7 Steps to Performance Enhancing ERM
This eBook highlights:
- 7 key trends in the “era of ERM”
- 6 characteristics of data-driven, performance-enhancing ERM
- The ERM process flow that will help you identify, respond, monitor, and manage risks, report on results, and continuously improve the process
- How to identify if you’re making common (and risky) ERM errors.
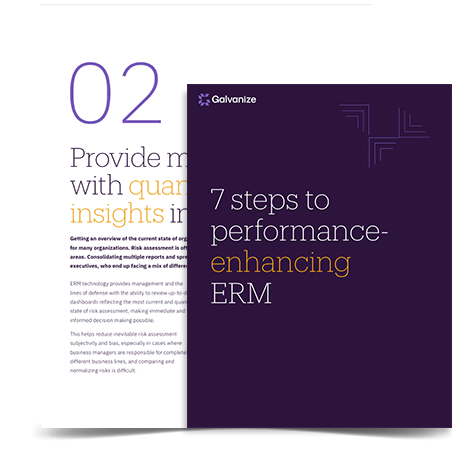