With fraudsters becoming more sophisticated, and global data increasing at exponential rates, data analytics is now, more than ever, a critical tool to deal with increasing levels of global fraud.
Did you know that fraud is costing organizations $2.1 trillion globally per year? That’s more than Brazil’s total GDP—the eighth largest world economy!
There’s no question that fraud schemes have become more sophisticated. Fraudsters are constantly finding new ways to manipulate technology. So, to combat fraud, organizations and governments around the world are heavily investing in technologies and resources.
In fact, a recent PwC survey found that 44% of respondents plan to increase spending on fraud prevention and economic crime over the next two years. The report says that most of this money is going toward advanced fraud detection software and data analytics and automation tools.
Data analytics are essential to detect and prevent fraud
The amount of data we produce worldwide is growing—and there’s no sign of it slowing. This makes it really hard to uncover fraud indicators. Internal controls are, on their own, not enough. (And employees are getting more cunning when it comes to finding ways around them.)
Manually reviewing all of this data is super costly and time-consuming—and simply impossible for large global organizations. However, with data analysis, you get a quick overview of your business operations and can easily drill down into details. This makes examinations much faster, more detailed, and more comprehensive than manual processes.
Benefits & applications of data analytics for fraud
- Automatically search 100% of your transactions for fraud indicators.
- Easily merge, normalize, and compare data from different systems.
- Quickly identify fraud before it becomes front-page news.
- Realign resources to focus detection efforts on suspicious transactions.
- More accurately calculate the impacts of fraud.
- Significantly reduce sampling errors and improve internal controls.
- Save time by automating repetitive tests.
Sampling is no longer good enough
There are some serious shortcomings with many controls testing methods like sampling.
- You can’t fully measure the impact of control failures.
- You can miss many smaller anomalies—which can result in very large frauds over time.
- Sample testing doesn’t find warning patterns or fulfill regulatory needs.
Although testing a sample of data is a valid audit approach, it’s not as effective for fraud detection purposes. This is because fraudulent transactions don’t generally occur randomly.
To effectively test and monitor internal controls, organizations need to analyze all relevant transactions—something that’s almost impossible to do without data analytics and automation.
“Proactive data monitoring was associated with 52% lower losses and frauds detected in half the time.” —Association of Certified Fraud Examiners
Types of analysis testing
Primarily, there are two types of analysis testing: ad hoc and repetitive/continuous.
Ad hoc testing
The goal of ad-hoc testing is to get an answer to a specific business question. Ad-hoc testing lets you explore and investigate your data. You can look into transactions and see if there’s anything to indicate fraud has occurred, or to identify opportunities for fraud to happen.
But this is still really manual and time-consuming. And, if that sort of anomaly seems to be relatively prevalent or there’s certain exposure to risk that you’re not comfortable with, maybe you want to investigate on a recurring basis, which leads us to the second type of testing.
Repetitive/continuous
Repetitive or continuous analysis for fraud detection means setting up scripts to run against large volumes of data to identify anomalies as they occur. This method can really improve the overall efficiency, consistency, and quality of your fraud detection processes.
Automating testing on obvious problem areas like P-Cards frees up your team to investigate other areas where things could be going wrong, or to focus on those tasks and projects that require a lot of time and manual attention.
“The Association of Certified Fraud Examiners reports that the typical fraud case goes on for 16 months before it’s detected.”
Progressing to continuous monitoring
There are obvious advantages to detecting fraud quickly, and timely risk mitigation makes a strong business case for analyzing and testing transactions on an ongoing basis.
Once a test has been developed to uncover a specific fraud indicator, it makes sense to repeat the analysis on a regular basis. How often you run the test depends on your goals and the size of your organization.
For example, in the case of monitoring payment and revenue transactions, it might make sense to perform automated testing on a daily basis. For areas like P-Cards, travel and entertainment (T&E) expenses, and payroll, you may only need to perform testing on a weekly or monthly basis, to align with payment frequencies.
It’s pretty straightforward to move from using a suite of fraud-specific data analytics on an ad-hoc basis to continuous monitoring. Assuming the issues of data access, preparation, and validation have been addressed—and that the tests have been proven effective—moving to continuous monitoring simply involves automating your testing. Then you can also set up an automated workflow for remediation.
Exceptions generated by specific tests will be automatically routed to specific individuals for review. Notification of high-risk exception items can be routed to more senior management. So, you can be sure issues are being flagged and followed-up on.
Getting started with data analytics for fraud
This is just an introduction to how data analytics can help with fraud detection and prevention. For more depth on the topic, including how to set up a fraud analytics program, the different types of analysis, and 20 common tests, check out our eBook, Detecting & preventing fraud with data analytics.
eBook:
Detecting & preventing fraud with data analytics
This eBook covers:
- Key considerations for implementing a successful fraud program.
- The most effective data analysis techniques for detecting and preventing fraud.
- Practical analytics tests you can implement right now across different business areas.
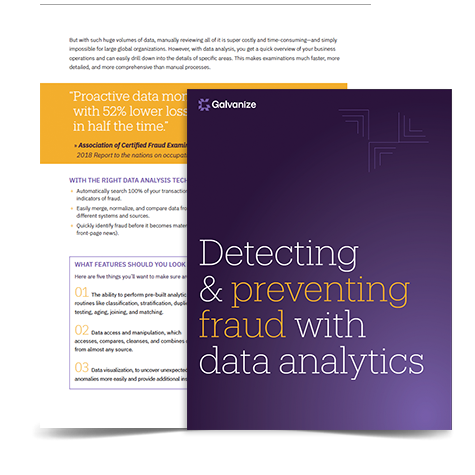