Machine learning now plays a huge role in helping organizations analyze their structured and unstructured data, identify new risks, automate manual tasks according to set triggers, and make data-driven decisions. In best-case scenarios, it can replace huge amounts of manual labor with automation, and provide insights that lead to better decisions around assessing, monitoring, and mitigating risk.
While machine learning is a risk management tool, it also poses many risks itself. While 49% of companies are exploring or planning to use machine learning, only a small minority recognize the risks it poses. And only 41% of organizations in a global McKinsey survey say they can comprehensively identify and prioritize machine learning risks.
This is why we wanted to showcase some of the risks of machine learning—and how they can be adequately assessed and managed.
What are the risks of machine learning data?
Poor data
Your machine learning model can’t grasp the context of the tasks it performs. It relies on human-supplied training data to work. (“Garbage in, garbage out” is often used to describe this issue.) Examples of so-called “dirty data” include errors in the data, outliers (such as a number with a significantly different value from other numbers, which can throw off averages), and unstructured data that can’t be adequately interpreted by the model (“noise”).
Overfitting
In overfitting, your training data fits the model so perfectly that there is not enough variability for the algorithm to learn from. That means it won’t be able to generalize when it comes to testing real data.
Biased data
Biased data means that human biases can creep into your datasets and spoil outcomes. For instance, the popular selfie editor FaceApp was initially inadvertently trained to make faces “hotter” by lightening the skin tone—a result of having been fed a much larger quantity of photos of people with lighter skin tones. If diversity and inclusion isn’t taken into account in your initial training data, it’s likely to show prejudices in the testing outcome.
Other types of machine learning risks
Beyond problems with the algorithm itself due to improper training sets, many organizations face other problems in implementing machine learning technology. These can include:
- Lack of strategy and experience
You’re bound to face a learning curve whenever you bring in new technology. But when it comes to machine learning, one of the biggest risks centers around the user’s experience—or lack thereof. A survey of over 2,000 people across a wide range of industries said a lack of a clear strategy (43%), followed by a lack of talent with appropriate skill sets (42%) were the biggest barriers to the adoption of machine learning. Without a strategy or the appropriate skill sets, you’ll be wasting time and resources on a solution that might not work—or one that might work, but in a way that could do damage to your organization. - Security vulnerabilities
If an outdated data source is included in your model, it may introduce security vulnerabilities into your organization by providing poor intelligence. - Regulatory challenges
If your team doesn’t understand exactly how an algorithm made a decision, they may lack the knowledge to justify decisions to regulators. - Third-party risks
One of your third-party providers may fail to properly govern a machine learning solution, leading to a data breach.
How to assess machine learning risks
That said, none of these risks make it unwise to incorporate machine learning into your organization. It simply means that it’s important to be methodical about how such technology is implemented, and to ensure that you develop a framework for managing the solution that’s put into practice throughout the organization.
To assess and manage the risks that your organization may face when implementing a machine learning solution, take these steps:
- Implement a risk management framework specifically for machine learning, rather than relying on a standard risk management framework that may not encompass the scenarios that you’ll face
- Educate the entire organization on how to follow protocols for effective risk management in machine learning
- Develop assessment criteria to help you prioritize risk focus across the business
- Reassess your risk profiles and risk appetites regularly, based on feedback from stakeholders throughout the organization
By adopting strong protocols and getting the right talent, strategy, and skills in place, you’ll be able to identify and prioritize machine learning risks and be in the position to take full advantage of this amazing technology.
Learn more
Machine learning offers endless potential for your organization, but its implementation and management must be carefully controlled to ensure that it doesn’t introduce new risks. Find out more about how to properly manage and mitigate the risks of machine learning in our new eBook.
eBook:
A risk-based approach to machine learning
Discover the role of risk identification, assessment, and management in machine-learning applications and how to:
- Identify, assess, and manage common machine learning risk.
- Use machine learning to support risk assessments.
- Find fraud with machine learning.
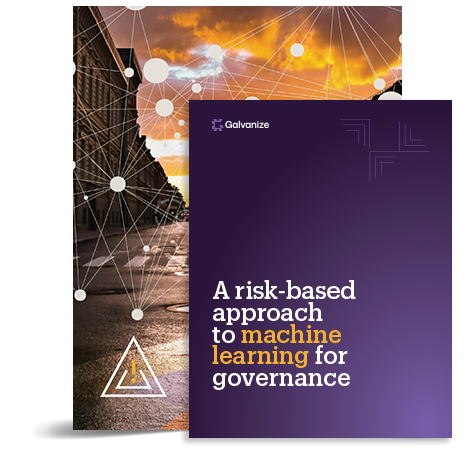